6.1. In context¶
This section considers the important area of latent variable modelling. These models have been shown, about 20 to 30 years ago, to be very powerful tools in dealing with the very data that (chemical) engineers face frequently. Our main goal of this section is to show how one can extract value from these data. But we first introduce the concept of a latent variable, and specifically the principal component analysis (PCA) model: the cornerstone of all latent variable models. Then we consider different ways to use our databases for interesting applications such as troubleshooting, soft-sensors, process monitoring, and new product development.
6.1.1. What we will cover¶
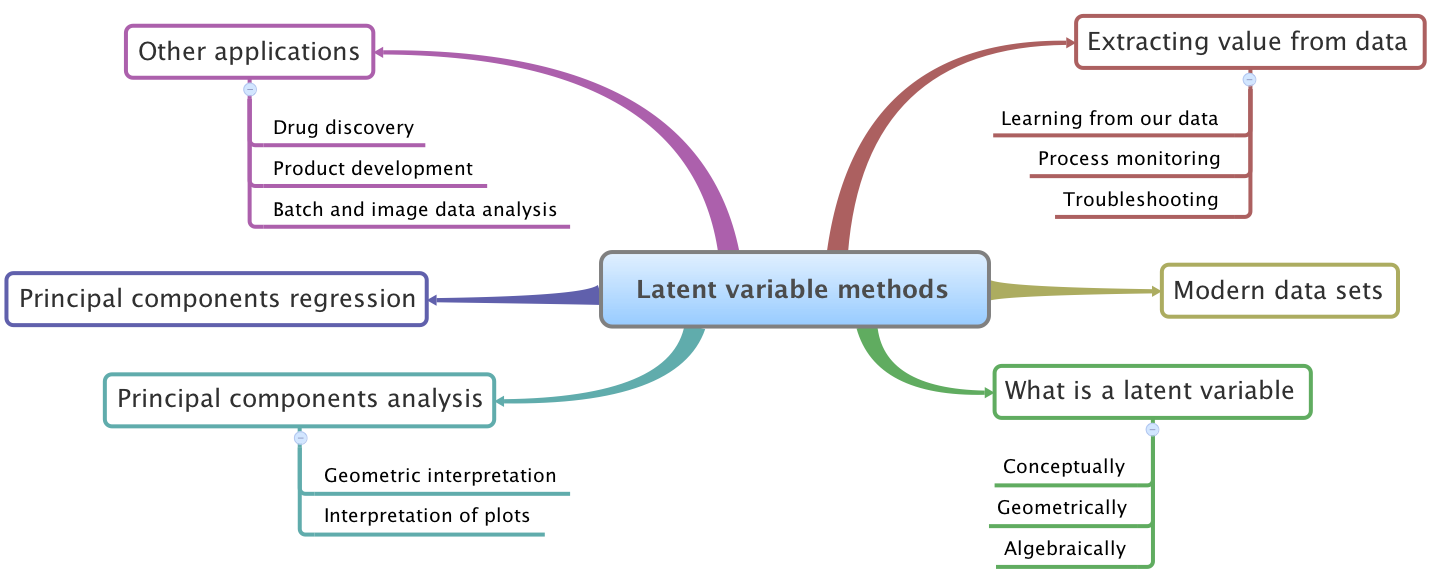
6.2. References and readings¶
These readings cover a variety of topics in the area of latent variable methods:
General: A collection of important latent variable publications are collected at https://learnche.org/literature
General: John MacGregor, Honglu Yu, Salvador García-Muñoz, Jesus Flores-Cerrillo, “Data-Based Latent Variable Methods for Process Analysis, Monitoring and Control”. Computers and Chemical Engineering, 29, 1217-1223, 2005.
General: Ericsson, Johansson, Kettaneth-Wold, Trygg, Wikström, Wold: “Multivariate and Megavariate Data Analysis”.
About PCA: Svante Wold, Kim Esbensen, Paul Geladi: “Principal Component Analysis”, Chemometrics and Intelligent Laboratory Systems, 2, 37-52, 1987.
PLS: Svante Wold, Michael Sjöström, Lennart Eriksson: “PLS-regression: A Basic Tool of Chemometrics”, Chemometrics and Intelligent Laboratory Systems, 58, 109-130, 2001.
PLS: S. Wold, S. Hellberg, T. Lundstedt, M. Sjöström and H. Wold, “PLS Modeling With Latent Variables in Two or More Dimensions”, Frankfurt PLS meeting, 1987 (available on request, by email to kgdunn@gmail.com)
PLS: Paul Geladi and Bruce Kowalski, “Partial Least-Squares Regression: A Tutorial”, Analytica Chimica Acta, 185, 1-17, 1986.
PLS: Paul Garthwaite, “An Interpretation of Partial Least Squares”, Journal of the American Statistical Association, 89, 122-127, 1994.
Process monitoring: John MacGregor and Theodora Kourti “Statistical Process Control of Multivariate Processes”, Control Engineering Practice, 3, p 403-414, 1995.
Process monitoring: J.V. Kresta, T.E. Marlin, and J.F. MacGregor “Multivariate Statistical Monitoring of Process Operating Performance”, Canadian Journal of Chemical Engineering, 69, 35-47, 1991.
Contribution plots: P Miller, RE Swanson, CE Heckler, “Contribution Plots: a Missing Link in Multivariate Quality Control”, Applied Mathematics and Computer Science, 8 (4), 775-792, 1998. (hard to obtain, but available on request, by email to kgdunn@gmail.com)
Soft sensors: J.V. Kresta, T.E. Marlin, and J.F. MacGregor, “Development of Inferential Process Models Using PLS”. Computers and Chemical Engineering, 18, 597-611, 1994.
Industrial applications: Ivan Miletic, Shannon Quinn, Michael Dudzic, Vit Vaculik and Marc Champagne, “An Industrial Perspective on Implementing On-Line Applications of Multivariate Statistics”, Journal of Process Control, 14, p. 821-836, 2004.
Batch modelling and monitoring: S. Wold, N. Kettaneh-Wold, J.F. MacGregor, K.G. Dunn, “Batch Process Modeling and MSPC”. Comprehensive Chemometrics, 2, 163-197, 2009. (available from the author on request, by email to kgdunn@gmail.com)
Image analysis: M. Bharati, and J.F. MacGregor “Multivariate Image Analysis for Real Time Process Monitoring and Control”, Industrial and Engineering Chemistry Research, 37, 4715-4724, 1998